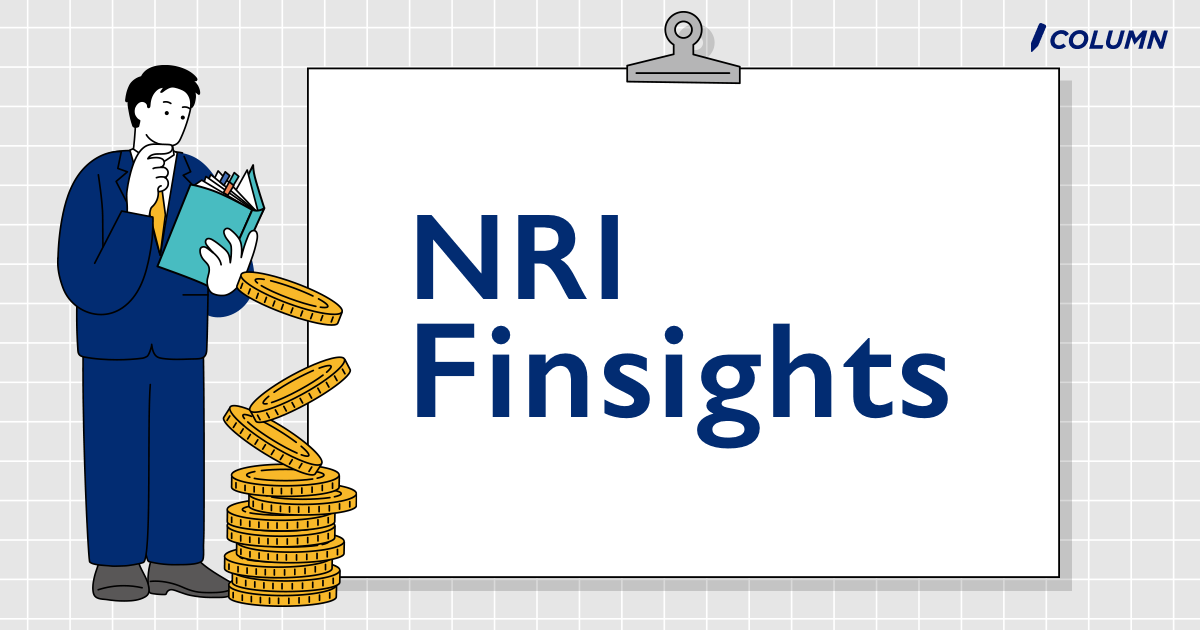
NRI Finsights
83 items
-
Trump Administration Announces Reciprocal Tariffs: 24% Additional Tariff Lowers Japan's GDP by 0.59%
-
[Case Study] NRI modernizes critical back-office solutions for brokerage firms with Oracle Cloud Infrastructure (OCI)
-
[Oracle CloudWorld 2024]: How Generative AI Solves Real-World Problems
-
[Featured on Digital Insurace]: A forecast of Gen AI in the Japanese insurance industry
-
The Bank of Japan's verbal intervention in the foreign exchange market: Caught between yen depreciation and stock price decline.
-
Dollar-Yen Rate Exceeds Defense Line: Foreign Exchange Intervention Looms
-
[Featured on Risk Management Magazine]: Conducting Effective Third-Party Risk Management
-
Japan January 2024 CPI (excluding fresh food)
-
The Persistent Headwind of Declining Real Wages: Domestic GDP Statistics for October-December 2023 to be Released
-
2024 US and Global Economic Outlook: Can AI boost a slumping world economy?
-
Will Governor Ueda’s Remarks Lead to Emerging Speculations Over Early Interest Rate Hikes?
-
Unrealized JGB Losses Growing Due to Higher Interest Rates, and Mounting Financial Risks in the Course of Policy Revisions
-
Japan’s July-September Quarter Shows Temporary Negative Growth
-
Understanding the capabilities of managed service providers
-
Will The BOJ Make Further Tweaks to YCC at its Next Meeting?
-
An Economic Policy Review of the Kishida Administration’s First Two Years: Further Promotion of Growth Strategies Under Continued Fiscal Consolidation a Problem Going Forward
-
Three Potential Scenarios Leading to a BOJ Rate Hike
-
[Featured on InsuranceNewsNet] Japan’s changing insurance industry can learn some lessons from the U.S. market
-
BOJ Rate Hike Speculations Lead Long-Term JGB Yields to Rise, Halt Yen’s Depreciation
-
[Featured on Traders Magazine]: Turning T+1 Worry Into Opportunity
-
Is a foreign exchange intervention to halt the yen’s depreciation coming soon?
-
The Inflation Rate Is Slowly Coming Down, But Rising Gasoline Prices Are A Cause For Concern (July Nationwide CPI)
-
Governor Ueda Hints at Putting Off Changes to Monetary Policy
-
Is the U.S. Inflation Crisis Over? (June CPI)
-
Are Historic Price Surges Showing Signs of Ending?
-
Why Does Yield Curve Control (YCC) Need a Revision?
-
With the US Debt Ceiling/Default Problem, Financial Market Turmoil and Public Opinion Trends Will Be Key
-
Silicon Valley Bank Failure Causes Increased Risk Aversion in Global Financial Markets: Speculation Over Suspension of U.S. Interest Rate Hikes Also Emerges
-
Challenges for the Next BOJ Administration(2): A Study of Kazuo Ueda
-
Challenges for the Next BOJ Administration(1): New Governor Ueda to Carefully Review Extraordinary Monetary Easing Framework
-
Kazuo Ueda reported to be next BOJ governor
-
Normalizing Monetary Policy and Communications at the Same Time is a Must for The Next Bank of Japan Governor
-
Amamiya reportedly vetted for BOJ governor post
-
The April Monetary Policy Meeting Becomes a Primary Focus
-
Financial markets awaiting BOJ’s next move and fresh inflation data
-
Was BOJ’s YCC adjustment a rate hike or not?
-
BOJ’s surprise decision to widen YCC band: outlook for revision of inflation target and joint statement still highly uncertain
-
What’s behind Japan’s unusual FX intervention?
-
Lack of economic dynamism is not to blame for weak yen
-
[Featured on Traders Magazine]: Capital Markets Are Changing, Are You Ready?
-
[Featured on GreenBiz]: How Japan is becoming a responsible investment success story
-
Kishida Government’s economic policy following LDP’s big win in Upper House election
-
<The BOJ’s ETF exit conundrum>
Part 5: BOJ’s ETFs could play a role in plan to double households’ asset-based income -
<The BOJ’s ETF exit conundrum>
Part 4: Will ETFs end up staying on BOJ’s balance sheet? -
<The BOJ’s ETF exit conundrum>
Part 3: Is there enough institutional demand for ETFs to absorb BOJ’s holdings? -
<The BOJ’s ETF exit conundrum>
Part 2: Shimoda proposal solves problem of how to fund government purchase of BOJ’s ETFs -
<The BOJ’s ETF exit conundrum>
Part 1: Freeze on ETF purchases as first step -
Liberal Arts Education
-
BOJ extension of COVID-19 operations highlights policy gap with other central banks
-
Art and technology
-
The diversification of human resources
-
Incoming Kishida Government’s expected economic policies
-
Emilio Greco and NRI
-
Potential economic losses from cancelation of Tokyo Olympics
-
The Arrival of the Biden Administration and the Negative Legacies of the Outgoing Trump Administration
-
VIDEO:Yasuki Okai - COVID-19 pandemic accelerates "Paradigm Shift" in Japanese financial industry
-
What Flaws Might Lie in the Substantial U.S. Economic Stimulus Measures for Supporting Private Businesses?
-
Tokyo Olympics Postponed; Severe Recession to Follow?
-
Why the being on the blockchain is not an excuse to forget your security standards
-
Financial Institution CIOs in Japan are Making Plans to Adopt AI Technologies
-
VIDEO: Takahide Kiuchi spoke to Beat Siegenthaler of the UBS Knowledge Network on Japanese monetary policy
-
The Challenges and Opportunities of Back Office Transparency
-
NRI and Thomson Reuters together are supporting Wealth Managers’ Seeking Greater Investment Opportunities
-
Thomson Reuters and NRI Announce Strategic Relationship
-
NRI Launches New Website
-
Coming Soon: Next Level Digital Assistants for the Financial Industry
-
What is a chatbot?
-
Thank you for visiting NRI's booth at 2017 Stockbrokers And Financial Advisers Conference!
-
The Back Office Disrupted: How Robotics and AI is transforming Operations within financial services
-
Your Operations are Full of Processes that You’re Paying Humans to Complete Inefficiently
-
Introduction to Cutter Associates, a new member of NRI Global Group
-
4 Things You Need To Know About RegTech
-
The Benefits and Challenges of NoSQL
-
Thank you for visiting NRI's booth at 2016 Stockbrokers Conference!
-
Thank you for visiting NRI at SIFMA Ops 2016!
-
What’s next in the US (50th anniversary blog 3)
-
Why US now (50th anniversary blog 2)
-
NRI's history in the US (50th anniversary blog)
-
Upcoming Regulation Changes in Japan in 2016
-
MEET NRI- Q&A with Yasuki Okai, President, NRI Holdings America
-
The Chief Data Officer (CDO)– New Kid in the C-Suite
-
Thank you for visiting NRI at SIFMA Ops!
-
Growth of ETFs and Their Infrastructure Needs